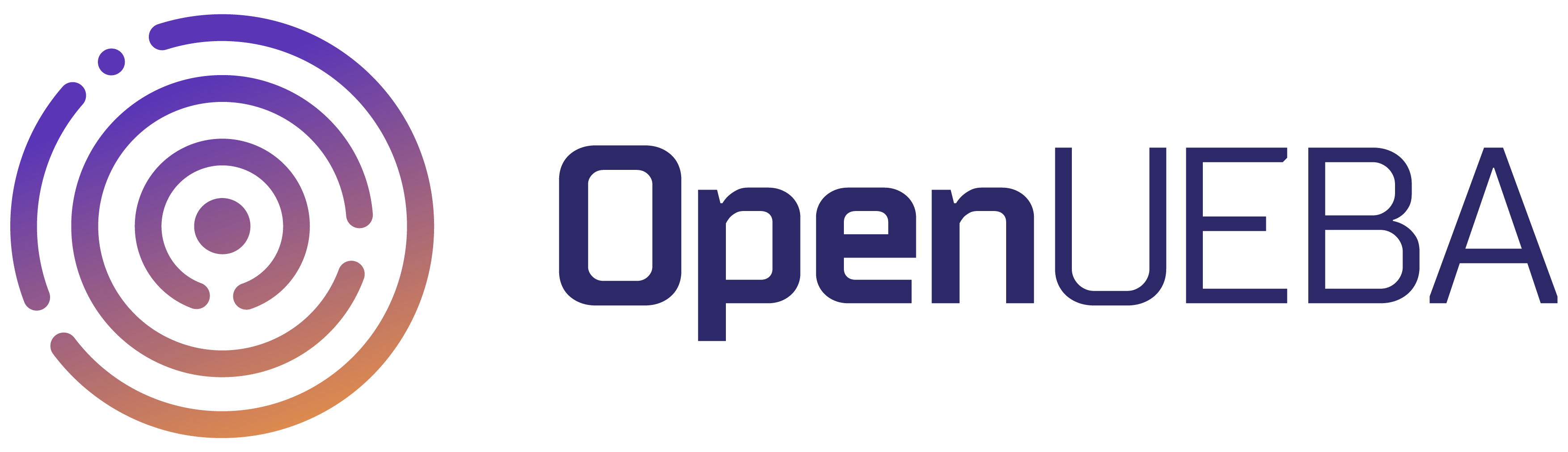
Behavioral Analytics – Artificial Intelligence for modeling the behavior of networked entities
Written by Albert Calvo – Research Engineer in Artificial Intelligence at the i2cat Foundation
Until now, security models against network incidents and threats are based on adding different layers of security to the infrastructure. We find the simile with the defenses of a castle; where the walls, the higher the better, allow the defense of the relics. Although, monolithic fortifications in digital environments have been an effective form of defense against fraud and digital threats; Today’s complex, hyper-connected and exponentially growing environment makes the tasks of protection, detection and response extremely difficult: the cyber security expert must monitor a large number of entities that generate large amounts of data per minute.
If we analyze the world of cyber attacks, we find a scenario of professionalized organizations and also with exponential growth. In fact, cyberattacks are already recurring news around the world, according to sources from the United States intelligence center, the number of attacks during the year 2021 increased by up to 150% compared to the year past being small and medium-sized businesses the main target of cybercriminals. Among the attacks that most affect companies and institutions are phishing attacks, where criminal groups impersonate users to obtain confidential user information. Ransomware attacks based on encrypting data and hijacking devices in order to demand rewards. Finally, denial of service attacks (Denial of Service) based on collapsing server resources, disabling the services it can offer.
In recent years, Artificial Intelligence (AI) has become a great ally in the fight against fraud and cyber threats in the digital world given the capabilities to optimize and automate the tasks of incident response services. Until now, AI techniques have been based on reactive threat detection, attack patterns are determined to detect future attacks, as for example in IPS systems where expert systems are trained to filter traffic potentially dangerous. Despite the effectiveness of reactive systems, these leave out the main factor of cyber threats: The human factor. Along these lines, research into user-centered techniques has gained importance in recent years; User and Entity Behavior Analytics (UEBA) techniques, where user behavior is analyzed through AI to offer both reactive and predictive capabilities towards latent attacks.
These techniques are based on studying the past, present and future behavior of the interactions of users and entities of a network, they allow to determine the normal and/or expected behavior of the entities of a corporation and any deviation in this behavior is analyzed through AI algorithms to search for and determine anomalous patterns or behavior resulting from cyber attacks. The main advantage of this type of techniques, compared to purely reactive ones, is the flexibility in the agnostic identification of threats, able to detect different types of attacks including zero-days and the early detection of threats: the detection in behavioral changes allow early stages of the attack vector to be detected.
The future trend is that cyber threats will continue to grow exponentially. We will experience more attacks, more diverse and affecting hitherto unimaginable institutions and entities. To deal with the predicted scenario, the UEBA techniques, previously described, will become a key point for the agnostic prevention of threats, helping to build cyber-secure digital environments.
In detail, UEBA techniques allow user behavior to be modeled based on two-dimensional analysis: historical and group components. In the first component, using artificial intelligence techniques, it is possible to profile the user and learn which patterns determine their behavior; using, for example, AI techniques such as decomposition into singular factors, clustering or state-of-the-art techniques based on deep learning (Deep Learning). On the other hand, the second dimension falls on systematically comparing the entities of the network to determine changes in behavior or anomalies, in this dimension, as in the previous case, there is a wide range of techniques, with clustering techniques being the preferred ones . Along these lines, the leading project in UEBA techniques, called OpenUEBA developed by i2cat, seeks to determine user behavior with the aim of calculating exposure to specific threats, helping the analyst to focus his efforts on those users with risk patterns that make it more likely to be affected by a specific threat.
References
- https://media.kaspersky.com/es/business-security/enterprise/Human%20factor_main%20threats_final_ES.pdf
- https://ciberseguridad.blog/ueba-user-and-entity-behavior-analytics-deteccion-por-comportamiento/
- https://blog.conzultek.com/ciberseguridad/inteligencia-artificial-en-la-ciberseguridad
- https://tdacyber.cat/
Published in CIDAI